Don’t invest unless you’re prepared to lose all the money you invest. This is a high-risk investment and you should not expect to be protected if something goes wrong. Take two minutes to learn more.
This article is not intended to be financial, investment or trading advice. This article is for information and solely for education purposes. It does not protect against any financial loss, risk or fraud.
Written by Lydia Rose Pallot
Topical ideas surrounding Artificial General Intelligence (AGI), including the vision that machines will be smarter than humans, have been the plot for sci-fi fictions for over a century. But, if we investigate predictions for the future, this is no longer fiction but reality.By 2029, machine intelligence is forecast to break out of specific tasks and into general intelligence. It is important to understand that these machines will be smarter than humans. By 2049, AI is expected to be at least one billion times more intelligent at all things compared to the smartest human.
Mo Gawdat, former Chief Business Officer of Google X, made a comparison of this intellectual gap in his book Scary Smart, by relating it to the intelligence of a fly in comparison to Einstein.This scenario, where humans become the fly in relation to machines, is hard to comprehend when we’ve long been ‘top of the food chain’. But, once we factor in machine intelligence superseding human intelligence, where would that place us?
There’s a huge array of questions surrounding AGI, the impact it’ll inevitably have on our everyday lives, our jobs, and the extent to which this innovation is accelerating. So, let’s first look at the current context in terms of growth, and then assess directly in relation to how it could impact the crypto space.
AGI. Slowly, then suddenly.
For some, AGI is a topic that has become more mainstream since the late 1990’s. However, AI has been a topic discussed amongst scientists dating back to 1950. Mathematician Alan Turing, famous for his role in cracking intercepted encrypted German messages in World War II, published a paper in 1950, Computing Machinery and Intelligence, where he introduced the now-famous Turing Test. Put simply, the Turing Test involved a natural language conversation between an evaluator, a human, and a machine. The machine was designed to generate a human style response. If the evaluator could not decipher between the human or machine response, the machine was deemed as having passed the test.
Shortly after, in 1956, a summer research project was held at Dartmouth College in the US, bringing together computer scientists who attempted to create early examples of AI. When I reference these dates, an easy mistake to make is to assume that because of the 67-year gap between 1956 and the present day (2023), the growth trajectory is slow and we’re another 30 years off AI becoming more mainstream. This couldn’t be more wrong. As with any technological innovation, AI needed funding to progress in the early development stages. 1973 was considered the first ‘AI winter’, where funding was pulled from various AI projects as the Middle Eastern oil crisis sent many economies into financial difficulty. Another AI winter occurred in 1987, triggered by another economic crisis. A turning point for AI came in 2009, when Google published a whitepaper introducing the concept of Deep Learning.
To summarise, the paper detailed an experiment where a machine was asked to ‘watch’ YouTube videos and observe patterns. No specific pattern for observation was requested;, the machine was left to its own devices. The first pattern it noticed was cats – clearly there’s a lot of cat content on the internet (which I fully support, cats are great). Once it had identified the features of a cat, it could easily find any video featuring a cat. Considering the vast number of videos on YouTube, for reference of scale we’re talking hundreds of millions, this is no small task.
Why this became a turning point is because shortly after having identified the cats, the machine applied the same approach to find words, foods, people, and endless other category types.
These neural networks are built through Deep Learning.
Following the breakthrough, AGI has accelerated in terms of both funding and progress. This growth is moving exponentially, as represented by the graph below.
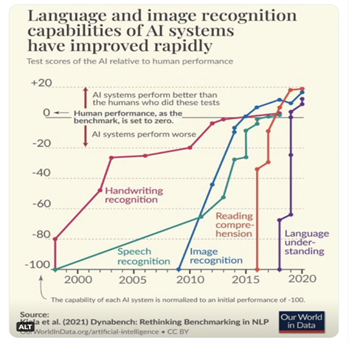
Blockchain and AI
With the understanding that AI and crypto aren’t mutually exclusive, we can look at how blockchain-based services are employing artificial intelligence and machine learning.
Operational efficiency
Though blockchain services aspire to decentralisation, there’s still a reliance on traditional business operations, which are being revolutionised by tools like ChatGPT. From copywriting to design, they’re increasingly being improved or replaced by AI.
AI could, for example, accelerate the construction of a metaverse or, through plugins, improve access to blockchain data, as Hedera Hashgraph, a decentralised public ledger platform, is already doing. Alongside the broader operational impact of AI on crypto businesses are a range of very specific applications.
Smart contract auditing
AI, like ChatGPT, is being employed to improve smart contract auditing using pre-training on datasets of known vulnerabilities, bugs and security risks. Auditing becomes faster and more cost-effective, which could reduce the risks of smart contract exploits.
Blockchain analytics
Blockchain analytics firms like Chainalysis leverage AI to help detect and prevent fraudulent activities. AI can also provide more meaningful analysis of user behaviour through network data from blockchain-based services and machine learning driven sentiment analysis of social media and forums. Santiment’s crypto intelligence tool is a good example of this.
Improved consensus mechanisms
AI algorithms can be used to optimise consensus algorithms and improve their efficiency, scalability, and performance.
Decentralised autonomous organizations (DAOs)
Just as AI can improve consensus mechanisms, it can play a role in decentralised autonomous organisations' (DAOs) governance and decision-making processes by analysing proposals, evaluating voting patterns, and providing insights to aid decision-making within these entities. For example, Aragon DAO is employing AI to understand more about its community, but the potential is for this to be scaled to analyse national elections and improve participation in democratic processes.
Predictive analytics
AI can be employed to produce and improve predictive analysis, informing trading and critical business decisions. Numeraire is an Ethereum-based marketplace for prediction models built on AI, while Ocean Protocol provides a blockchain-based data marketplace, including private data, against which to train models.
Verification & identity
AI could be combined with blockchain to add a unique digital signature for each piece of digital content, immutably stored on-chain. For example, Worldcoin is a blockchain-based identity protocol using biometric data to distinguish people from bots.
AI and possible threats to the crypto space
As with most technology, the potential of AI for the crypto space is a double-edged sword which, as a tool, can equally be used for harm.
Misinformation
Crypto’s speculative nature is the consequence of an absence of information. This lends itself to gossip and rumours moving the market, which is why pump-and-dump tactics can be so effective. The ease with which AI allows the creation of deep-fake videos could generate a tidal wave of misinformation, from spoofed influencer endorsements to fabricated news, with profit to be made from trading the impact on price.
More scams
AI reduces the barrier to entry for bad actors, from spinning up fake protocols and worthless tokens to market manipulation. Without guard rails, AI could facilitate an increase in crypto scams.
Increase smart contract hacks
Though AI is being used to improve smart contract auditing, it's as easy to exploit any vulnerabilities for profit as fix them. This may lead to an arms race in AI analysis and organised hacks, such as those by the Lazarus Group.
The relationship between crypto and AI: A summary
Overall, when we consider the role AI will play in our future, the impact on not just the crypto industry but also more widely is dependent on a range of factors. As I referenced at the start of this article, Gawdat outlines in Scary Smart that there are three ‘inevitables’ when it comes to AI:
- AI will happen.
- AI will be smarter than humans.
- Bad things will happen.
The scale at which these occur, and the severity of the potential positive or negative impact, is dependent on the current and future actions we take when it comes to AI. Considering that blockchain-based services are already employing artificial intelligence and machine learning, and the widespread adoption of tools such as ChatGPT, AI is already happening. The forecasts already show machines are smarter and becoming increasingly more intelligent than humans. And, as outlined in the potential threats to the crypto space, it isn’t hard to translate these on a wider economic scale when it comes to the risks.
Try crypto with Skrill today
- Buy and sell over 40 different cryptocurrencies
- Set conditional orders to automate your trades
- Earn points and be rewarded with Skrill's loyalty programme, Knect
Cryptocurrencies are unregulated in the UK. Capital Gains Tax or other taxes may apply. The value of investments is variable and can go down as well as up.
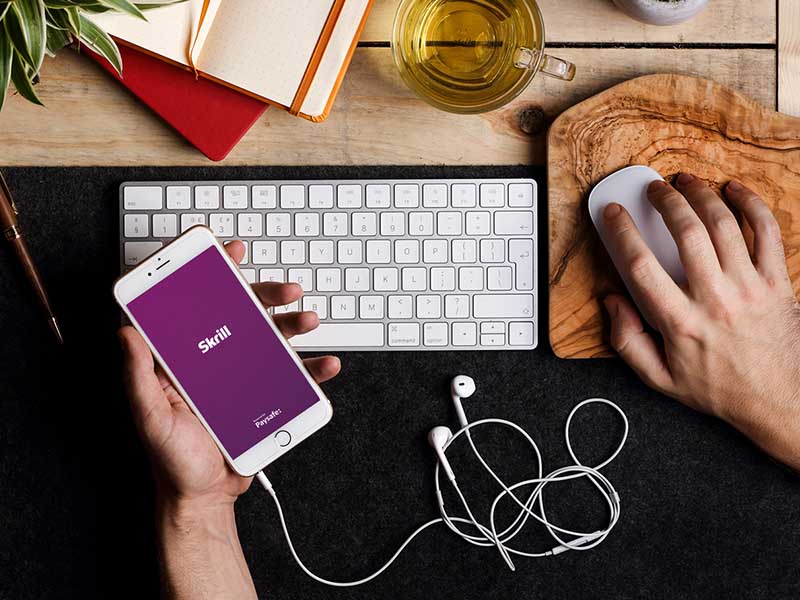